AI-Powered Demand Forecasting in a VUCA World: How to Reduce Auto Inventory Costs by 30–50% with Hybrid AI
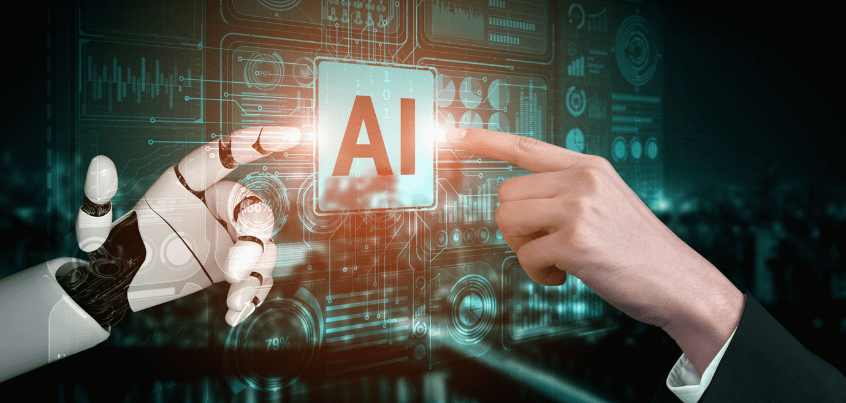
Automotive supply chains continue to operate under high uncertainty. From fluctuating EV demand and policy shifts to global supply disruptions and chip shortages, traditional inventory planning methods are falling short. While precision in manufacturing and logistics has improved, demand forecasting and inventory management often rely on static, rule-based models that fail to adapt to market dynamics.
The result is a costly imbalance: excess inventory that ties up working capital and critical stockouts that delay services and reduce customer satisfaction. The challenge is clear, but so is the opportunity for change.
This article outlines a hybrid AI approach to automotive demand forecasting, based on hands-on experience working with EV startups, legacy OEMs, and multi-tier suppliers. The methods discussed have helped reduce forecasting errors, improve part availability, and optimize working capital at scale.
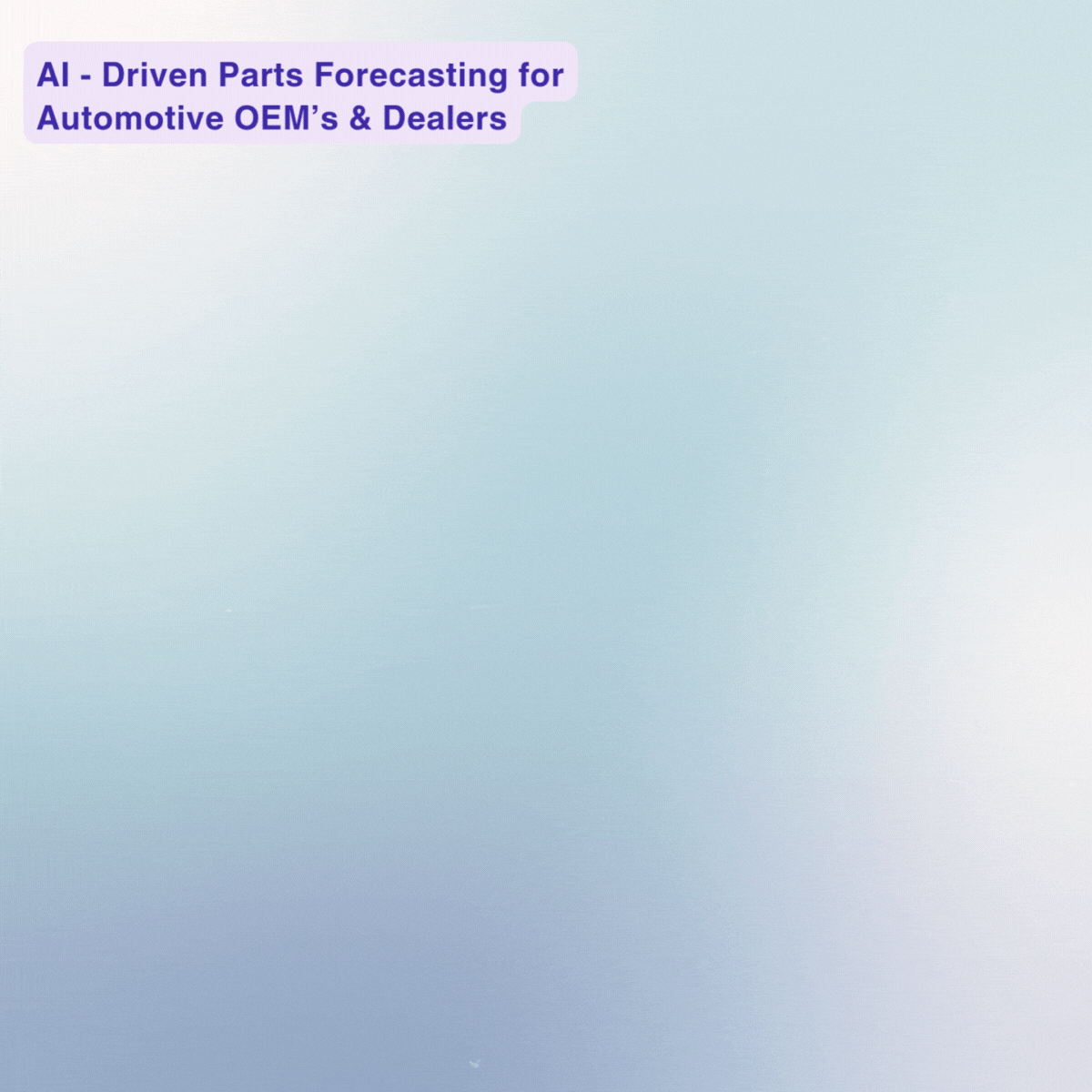
Why Inventory Management Still Has Gaps
Across OEMs, dealers, and suppliers, recurring problems surface when inventory isn’t aligned with real demand:
- Working capital is tied up in slow-moving inventory, often due to aggressive stocking strategies supported by OEM financing.
- Service delays occur when fast-moving parts run out, resulting in poor customer experiences.
- Parts availability is affected by delays and disruptions, especially in global supply chains.
- Traditional methods such as ABC classification or FMS planning rely on static rules and cannot respond to rapid market shifts.
These issues are not isolated. They point to deeper limitations in legacy forecasting systems.
Root Causes of Forecasting Inaccuracy
Several factors contribute to persistent forecasting gaps:
- Forecasting models show 30 to 40 percent error rates when compared to actual demand.
- Safety stock logic lacks the flexibility to adjust for real-world disruptions like semiconductor shortages or regional demand spikes.
- External signals such as economic trends, policy changes, competitor actions, and weather patterns are often excluded from planning logic.
The financial consequences are significant. From EV disruptors to established ICE brands, millions are lost annually in inefficiencies that are preventable with the right forecasting strategy.
A Practical Approach to Hybrid AI Forecasting
To address these challenges, we implemented a forecasting system that combines statistical modelling, reinforcement learning, and external event intelligence. The following steps outline the approach and its real-world outcomes:
Step 1: Model Baselining with Advanced EDA
Each engagement begins with a comprehensive exploratory data analysis. This step identifies demand variability, historical trends, and forecast gaps across SKUs and geographies.
A model library of over 20 time-series and reinforcement learning models—including SARIMA, Prophet, and customized RL agents is evaluated during onboarding. Early implementations have shown a baseline accuracy improvement from 60–70 percent to 85–95 percent.
Step 2: Dynamic Model Selection
Rather than locking into a single model, the system selects the most accurate one for each forecasting cycle. This dynamic selection process evaluates model performance weekly or monthly, ensuring that short-term shifts in market behaviour are captured in real time.
Step 3: Reinforcement Learning for External Events
Real-world demand is heavily influenced by external events, which legacy systems fail to capture. Our RL layer integrates human input with autonomous learning to account for dynamic signals.
Key features include:
- Planned Event Inputs: Planners can input known events such as regulatory updates, elections, or subsidy launches.
- Event Impact Estimation: Using contextual multi-armed bandits, the system estimates how each event will affect different categories or regions.
- Temporal Pattern Learning: A growing database of temporal event-response patterns helps the system understand how demand changes before, during, and after major events.
- Similarity Matching for New Events: When new events occur, vector similarity techniques match them with previously seen patterns to suggest forecast adjustments.
- Planner Feedback Loop: Forecast suggestions can be reviewed and adjusted by planners. The system learns from these decisions to improve future recommendations.
- Event Intensity Scoring: By combining news sentiment, historical data, and economic signals, each event is scored to reflect its likely influence on demand.
Step 4: Auto-Retraining and Drift Detection
Model performance is continuously monitored using statistical drift detection. Forecasts are automatically retrained when data patterns shift, eliminating the need for manual recalibration.
- Volatile markets, such as those involving new EV models, follow a six-month drift window.
- Mature demand environments typically operate on nine to eleven-month retraining intervals.
This allows the system to remain accurate without overfitting to short-term noise.
Step 5: Short Forecast Horizons for Greater Precision
Forecasting is kept within a one to three-month window to ensure model relevance. Longer horizons are avoided, as they often extend beyond the valid range of training data and event patterns.
Real-World Business Outcomes
Across multiple automotive segments, the system has demonstrated measurable business improvements:
Use Case | Forecast Accuracy Improvement | Operational Benefit |
---|---|---|
EV OEM | 30% → 6% prediction error | Improved availability of fast-moving parts |
ICE Automaker | 50% inventory optimization | Higher working capital efficiency |
Dealer Network | 40% stockout reduction | Faster service turnaround and improved customer satisfaction |
Why This Works
- Forecasting accuracy is up to six times higher than rule-based systems.
- The system adapts autonomously, reducing manual intervention.
- Every forecast recommendation is explainable, helping supply chain teams understand the rationale behind adjustments.
Conclusion
The automotive industry demands precision at every level. Yet inventory planning often relies on outdated systems that struggle with today’s pace of change. A hybrid AI(1) approach brings flexibility, accuracy, and explainability into forecasting, enabling companies to reduce waste, improve service levels, and free up working capital.
At Excellon, we’ve been working with OEMs, EV disruptors, and dealer networks to build forecasting systems that are not only intelligent, but grounded in the realities of automotive operations. If your team is facing similar challenges, we’d be glad to share what’s worked in our experience.
Frequently Asked Questions
How long does it take to train and deploy the models?
Training timelines range between six and eleven months, depending on market volatility. Forecasts are generated in real time using the most suitable model at each cycle.
Will this replace human planners?
No. The system supports planners by simplifying complex calculations and flagging high-risk patterns. Final decisions remain in the hands of experienced teams.
Is this approach viable for smaller networks?
Yes. The solution can scale from single-site dealers to large OEM ecosystems, with customizable deployment models.
Looking Ahead: From Forecasting to Fully Autonomous Supply Chains
As automotive supply chains evolve, the next frontier includes:
- Generative AI for running scenario-based simulations, helping planners prepare for disruptions.
- Integrated bots that connect forecasts with sourcing decisions, automating supplier communications and negotiations.